Computational medicine
Computational Imaging and Simulation Technologies in Biomedicine
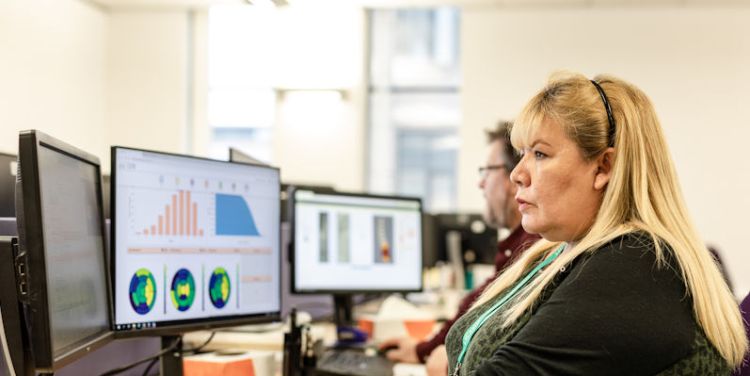
The Center for Computational Imaging and Simulation Technologies in Biomedicine (CISTIB) is an interdisciplinary centre that conducts research in computational medicine, spanning the School of Computing and The Faculty of Medicine and Health.
Computational Medicine (in-silico Medicine) applies methods from computational sciences, engineering, and mathematics and to improve our understanding and treatment of human diseases. Our group performs cutting-edge research in areas of fundamental and applied biomedical image analysis and modeling with impact in personalised diagnoses, minimally invasive treatment and in silico clinical trials. We have an international profile and strongly commit to clinical and industrial translation with impact in future healthcare.
Our research
Our current research can be grouped into various areas:
Artificial intelligence in medical imaging
We develop novel AI methods capable of transforming rich medical imaging data in quantitative biomarkers with applications in computer aided diagnoses and patient-specific interventional planning. This provides quantitative information from individual patients so the best course of action in their care pathway can be chosen. Our group uniquely combines expertise core AI research with a genuine translational research working from clinical unmet needs to research on generic AI methods, and then to deliver innovative solutions to our clinical collaborators.
Related projects:
- BQ-MINDED – Breakthroughs in quantitative magnetic resonance imaging for improved detection of brain diseases
- EDIFICE – Early detection of cancer recurrence in patients with glioblastoma using artificial intelligence through imaging
- CRUK RadNet – Personalised and adaptive radiotherapy
Computational imaging phenomics
This involves working on the challenges open up by large-scale image databases like population imaging data (e.g. UK Biobank) or NHS-like care repositories. We develop AI methods robust and accurate to deal with the complexity and heterogeneity of imaging databases. We also are interested in novel methods to link imaging biomarkers with non-imaging data like omics and genetics.
Related projects:
- CARDIOMICS – Novel cardiac phenotyping for population imaging and imaging genetics
- BIANDA – Bayesian deep atlases for cardiac motion abnormality assessment from imaging and metadata
Patient-specific modelling and simulation
We go beyond phenomenological models (i.e. black-box models like ML or AI techniques). We also work on mechanistic models built on first principles on physics, physiology, biochemistry, etc. and inform them with healthcare data, notably medical images and physiological signals. This enables us to generate meaningful predictions from new data or unseen patient scenarios. These techniques are particularly relevant for patient-specific prediction of treatment alternatives.
Related projects:
- INSILC – In-silico trials for drug-eluting bioresorbable vascular scaffold design, development and evaluation
In silico clinical trials for medical devices
This focuses on creating virtual populations of patients based on advanced medical imaging data and novel statistical methods allowing us to model the variability of anatomical and physiological conditions across a population. We also can incorporate uncertainty modelling and propagation to understand how modelling assumption and errors or natural inter-subject variability affects the robustness of a given device design.
Related projects:
- INSILEX – Computational precision medicine for in-silico trials of medical devices
Massive-scale health data sharing, modelling and simulation platforms
Over the years, we have developed unique capabilities and infrastructures on cloud-based eco-systems facilitating running massively large image-based analytics or image-based biomechanical simulations. For example, models and simulations of individual patient physiology under uncertainty in modelling assumptions or experimental data to in silico clinical trials of medical devices on virtual populations.
Related projects:
BACKUP – Personalised prognostic models to improve well-being and return to work after neck and low back pain
MULTI-X – A cross-domain research-oriented platform for accessible, collaborative and reproducible computational and data-intensive analysis
Collaborations and partnerships
We collaborate extensively with other parts of the University, and with universities and companies across the world. Ongoing collaborations include:
Contact us
View all members of our research group, our recent projects and publications.
If you are interested in collaborating with us or joining our research team, please contact Professor Alejandro Frangi, Dr Zeike Taylor or Dr Toni Lassila.
PhD projects
We have opportunities for prospective postgraduate researchers. Find out more.
We also have 10 places each year in the UKRI Centre for Doctoral Training in Artificial Intelligence for Medical Diagnosis and Care (CDT).
Unless you are applying for a funded project or the CDT, you should start out by finding a supervisor .
News and events
Follow us on Twitter for the latest updates.