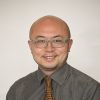
Dr Xiaohui Chen
- Position: Associate Professor
- Areas of expertise: Materials and Fluids, Machine Learning, Geotechnical Engineering, Multiphysics Modelling, Environmental Engineering, Water Science, Sustainable Agriculture and Food, Biodiversity.
- Email: X.Chen@leeds.ac.uk
- Phone: +44(0)113 343 0350
- Location: 4.04 School of Civil Engineering
- Website: Geomodelling and AI Group | Googlescholar
Profile
Dr. Chen is an Associate Professor at the University of Leeds, specializing in Physics-Informed Machine Learning (PIML) and Coupled Multiphysics Modelling (e.g., Mixture Coupling Theory) for Materials and Fluids. His research has broad industrial applications, including geotechnical engineering, water research, subsurface disposal, energy, sustainable agriculture, and environmental engineering. Additionally, he is a Fellow of the Institution of Environmental Sciences and a Chartered Environmentalist (UK).
Geomodelling and Artificial Intelligence Group: Dr. Chen is the founder and director of the Geomodelling and Artificial Intelligence Group, which includes 2 postdoctoral researchers, 11 PhD students, and 9 MSc/MEng students. The group has secured over £2.7 million in funding from research councils (including NERC, EPSRC, Horizon EU, and CSC) and industry partners. This group focuses on cutting-edge theoretical, numerical, mathematical, and AI modeling, with proven impacts in various industries such as petroleum, nuclear waste, carbon capture and storage, landfill, tunneling, water, and agriculture. Collaborating with world-renowned academics and institutions, the group aims to achieve low-carbon, long-life infrastructure, circular economy, and environmental protection, mitigating the impact of climate change on human civilization..
Selected Recent Invited Research Seminars/Presentations/Keynote Speakers:
- Founder and Chair of Physics-Informed Machine Learning (PIML) International Seminar, February 2024, TC309, ISSMGE.
- University of Cambridge, Geotechnical Engineering Seminar, November 22, 2023. [Link to seminar details]
- Ecole des Ponts ParisTech (ENPC), Geotechnical Engineering Seminar, Paris, France, August 2023.
- The Norwegian Geotechnical Institute (NGI), Research Seminar, Oslo, Norway, August 2023.
International Standing:
- UK representative on the International Society of Soil Mechanics and Geotechnical Engineering (ISSMGE) Technical Committee (TC309) Machine Learning and Taskforce Leader on Physics-Informed Machine Learning (PIML)
- UK representative on the International Society of Soil Mechanics and Geotechnical Engineering (ISSMGE) Technical Committee (TC215) Environmental Geotechnics
- Champion of a Themed Issue for ICE International Journal Environmental Geotechnics:“ Physical-Chemical Coupling in Environmental Geotechnics"
- Associate Editor of the Journal: Geomechanics and Geoengineering
- Editor Board: Environmental Geotechnics
Awards and Distinctions:
- Winner of Faculty (EPS) Partnership Awards 2022 for Academic Personal Tutor/Supervisor Award.
- "Top 5 Highly Downloaded Articles in 2013" from International Journal of Engineering Science (IF:9.052).
- Champion the themed issue of Environmental Geotechnics (2020-2021): “Physical-Chemical Coupling in Environmental Geotechnics”.
- Co-author of the "front cover" article from Environmental Science & Technology (IF:7.149).
- Solo author of an article from International Journal of Solids and Structures (IF:2.787).
- One of the founders of Tay-Gene Geotechnical Centre (2014-2015), Dundee.
- Fellow of Higher Education Academy, was nominated for a university teaching award of “most inspiration moments”, and contributed to increasing the satisfaction rate of students in Civil Engineering at UoA from 65% to 97% (NSS) (2015).
- ORS Award (2007-2010), and NERC PDRA within £3m BIGRAD consortium (2010-2014).
Publications Summary: Published over 70 articles in top-tier international journals, including
- Highly Cross-disciplinary Engineering: International Journal of Engineering Science (3, IF=9.219), Journal of Hazardous Materials (2, IF=9.038), Chemical Engineering Journal (1, IF= 13.273).
- Geotechnical and Geoenvironmental Engineering: Water Research (1, IF=13.4), Environmental Science and Technology (1, IF=7.864, Front Cover), International Journal for Numerical and Analytical Methods in Geomechanics (4, IF=4.621), Advances in Water Resources (1, IF=4.5), Journal of Hydrology (1, IF=4.5), Science of The Total Environment (1, IF=6.551), Journal of Geotechnical and Geoenvironmental Engineering (1, IF= 4.012), Computers and Geotechnics (4, IF= 4.956), International Journal of Solids and Structures (2, IF=3.9), Applied Thermal Engineering (1, IF=6.46), Journal of Geotechnical and Geoenvironmental Engineering (1, IF= 4.012), Journal of the Mechanics and Physics of Solids (1, IF=5.582).
Responsibilities
- Deputy Director of Research and Innovation
Research interests
My research has garnered funding from prestigious research councils such as EPSRC, NERC, Horizon EU and CSC, as well as from international industry leaders across the UK, EU, and Asia. Below is a selected list of grants:
- Horizon EU (UKPI): MARIE SKĊODOWSKA-CURIE ACTIONS Staff Exchanges (SE), Geotechnical Resilience through Intelligent Design. UK leads Physics-Informed Machine Learning
- NERC (Co-I): iCASP Mini Project: Duration: 2023-2024. Description: Prioritising River Habitat improvements for biodiversity net gain using machine learning. Funding: One 6-month PDRA is funded.
- EPSRC (PI): MSc Team Research Project: Duration: 2023-2024. Description: Flow behavior of and in bio-soils influenced by organic matter and mineralogy. Funding: Funded through EPSRC Centre for Doctoral Training in Fluid Dynamics (EP/S022732/1).
- EPSRC (PI): Temperature-water-plant-soil Coupled Modeling for Biogeotechnics: Duration: 2022-2026. Description: Case studentship jointly funded by industry partner N2-Applied. Funding: EP/W524372/1.
- H2020-EU (PI): BioGeoInterface: Duration: 2022-2024. Description: Grant agreement ID: 101031565. One Marie Curie Fellow was funded. Funding: Link to Project.
- CSC (PI): Physics-Informed Deep Learning for Quick Prediction of Geo-infrastructure Response to Climate: Duration: 2022-2026.
- NERC and Deltares Institute (PI): Using Deep Learning for Groundwater Prediction: Duration: 2021-2024 (NE/S007458/1).
- Strabag Tunnelling (PI): THMC Coupled Modeling for Tunnelling: Duration: 2021-2023.
- CSC (PI): Bio-inspired Penetrating and Burrowing Strategies: Duration: 2021-2024.
- N8 Universities Agriculture Food Funds (PI): Real-Time Moisture and Temperature Modeling for Sustainable Agriculture Against Extreme Weather-Induced Pest Attack: Duration: 2021.
- SUSTech + Leeds Funding (PI): AI for Surface and Groundwater Modeling: Duration: 2020-2024.
- Kuwait Oil Company (PI): THMC Coupled Modeling for Carbon Capture and Storage: Duration: 2019-2024.
- EPSRC IAA Phase 3 (Co-I): EP/R511717/1: Duration: 2018-2021.
- Shell & CSC (PI): THMC Modelling for Shale Gas: Duration: 2019-2023.
- Kuwait Oil Company (PI): Reactive Transport Modeling for Naturally Occurring Radionuclides: Duration: 2018-2022.
- White Rose University Consortium (PI): Sustainable Agriculture: Biochemical-Physical-Biological Function of Sludge in Agricultural Soils (BIOSAS): Duration: 2016-2020.
Qualifications
- PhD The University of Manchester, 2010
- MSc Dalian University of Technology, 2007
- BEng Dalian University of Technology, 2003
Professional memberships
- Fellow of The Institution of Environmental Science
- Chartered Environmentalist
- Fellow of Higher Education Academy
Student education
I presently teach:
- CIVE5162 (MSc Geotechnical Engineering) Constitutive Model and Numerical Analysis (Module Leader)
- CIVE5574/5575 (MSc EEPM and Geotechnical Engineering) Groundwater Pollution and Contaminated Land (Module Leader)
- CIVE3750 Individual Research Project
- CIVE5755 Individual Research Project
Research groups and institutes
- Water, Public Health and Environmental Engineering
Current postgraduate researchers
- Sulaiman Abdullah
- Yousef Baqer
- Y MA
- Jianting Feng
- Maria Luisa Taccari
- Paul R Howlett
- He Yang
- Jiahui Zhou
- Yanjie Song
- Biao Yuan
- Liyang Mao
Projects
-
<li><a href="//phd.leeds.ac.uk/project/906-coupled-thermo-hydro-mechanical-chemical-modelling">Coupled Thermo-Hydro-Mechanical-Chemical Modelling</a></li>
<li><a href="//phd.leeds.ac.uk/project/1133-physics-informed-deep-learning">Physics informed deep learning</a></li>
<li><a href="//phd.leeds.ac.uk/project/907-temperature-water-plant-soil-coupled-modelling-for-biogeotechnics">Temperature-water-plant-soil coupled modelling for biogeotechnics</a></li>