Geometrical digital twins of masonry structures for documentation and structural assessment
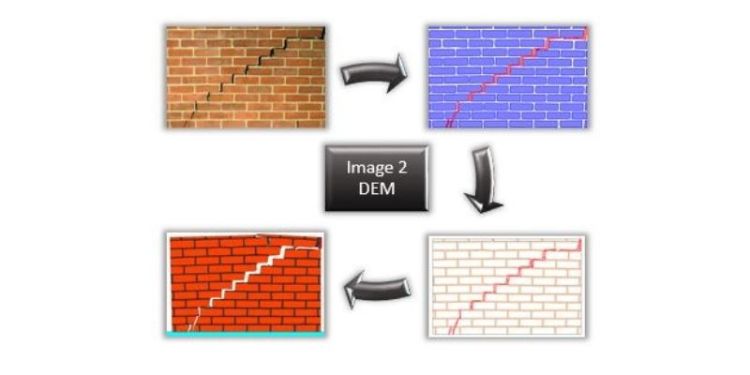
Researchers have developed algorithms capable of automatically generating the geometric digital twins of existing masonry structures for documentation and assessment of their structural capacity.
The models can be used to perform a detailed structural analysis – to assess, for example, any weak points or to make predictions about the expected lifespan of the structure.
Geometry of masonry infrastructures is typically captured with traditional techniques such as visual inspection and manual surveying methods, which are labour intensive and error prone. In the last ten years, advances in remote surveying methods, such as remote sensing and computer vision, have started to drastically change the building industry, since such techniques are able to accurately and remotely capture exact digital records of objects and features in a very short time with excellent accuracy.
The researchers from the School of Civil Engineering at the University of Leeds developed an applied workflow, where images captured from any source (i.e., smartphone, DSLR camera, drone etc.) are imported into our “Image2DEM” software developed to acquire the precise geometry of existing masonry structures. The developed software is able to detect the location of masonry units (i.e., bricks, stone), and perform damage assessment, using, state-of-the-art, artificial intelligence and image-processing algorithms.
This process fully automates the “scan-to-damage-assessment” and “scan-to-structural-analysis” procedures. The workflow of the developed program is presented in the image above.
- An image is captured using either a DSLR or a smartphone. Any image from any source can be used. Images that include any background (random objects, sky, ground, etc) are also compatible. Orthorectified images (with equal height/width scale) and good resolution are preferred but are not necessary.
- The image selected is then imported to the Image2DEM software to detect and extract the masonry micro-geometry. The algorithm provides reliable detection using artificial intelligence. Convolutional Neural Networks (CNN) are used to identify the location of masonry units and cracks, with ~96% and ~80% accuracy, respectively. Furthermore, background elements (non-masonry) are filtered out automatically.
- The geometry extracted is converted to a numerical model for analysis. Furthermore, the mesh generated allows the investigation of separation (loss of contact between structural elements) during the analysis. To enhance simplicity, the elements are allocated to different groups depending on the layers assigned on the CAD file.
- The geometry is extracted in the form of simplified lines to improve efficiency and reduce computational effort and a “geometric digital twin” is created. Blocks, mortar, and cracks are assigned to different layers automatically. The mesh is optionally generated for blocks, mortar, and cracks. The output is provided in DXF format for compatibility between different computer aided design (CAD) programs.
Mr. Dimitrios Loverdos and Dr. Vasilis Sarhosis carried out the research.
“This transition from the physical to the digital environment has the potential to gain a better understanding of the “as is” condition of our existing masonry infrastructure and revolutionise the way structural analysis is performed in industry.”
The aim is to provide detailed and accurate data that will better inform maintenance programmes and asset management decisions in relation to our ageing masonry infrastructure stock.