Detecting masonry cracks with artificial intelligence
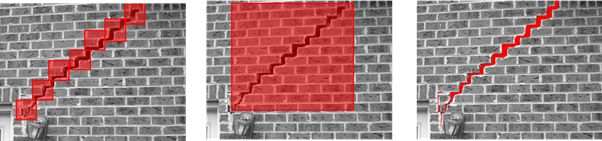
Researchers have, for the first time, developed algorithms based on AI and deep learning that are able to trace cracks in masonry from digital images.
Researchers have, for the first time, developed algorithms based on AI and deep learning that are able to trace cracks in masonry from digital images.
Dr Vasilis Sarhosis and Mr Dimitris Dais from the University of Leeds and Professor Ihsan Bal and Dr Eleni Smyrou from Hanze University of Applied Sciences in Netherlands, led the research. They are part of an international team of engineers and scientists researching infrastructure of the future.
Masonry structures represent the highest proportion of building stock worldwide, and cracking is the most frequent cause of masonry performance loss and has been an engineering concern for centuries.
Currently, the structural condition of masonry structures is predominantly manually inspected, which is a laborious, costly and subjective process. Manual inspection is also problematic since there are often parts of structures with access restrictions or are simply difficult to reach. Manual inspection is also particularly difficult for ‘post-event’ cases, such as in the catastrophic aftermath of a strong earthquake, when a high number of buildings need to be inspected with limited resources in a short time.
Using developments in computer vision, a field of artificial intelligence that trains computers to interpret and understand the visual world, the research team used digital images to automate the structural visual inspection process.
Dimitris Dais, Civil Engineering PhD Researcher, said: “It was mesmerising to see the machine becoming smarter and smarter and indeed learning to classify a wider range of crack types by providing additional data and using suitable AI models”.
Starting with a dataset containing photos from masonry structures containing cracks, deep learning networks were developed to classify images from masonry. The approach achieved a high level of accuracy in identifying images where cracks were present, which demonstrates the potential of the approach.
The proposed research has the potential to revolutionise the way we assess our existing masonry infrastructure in the future and contribute towards network resilience and infrastructure sustainability not only in the UK but internationally.
Cracks were not only registered correctly by using the technique but their measurements - crack length and width – were also automatically obtained.
Dr Sarhosis, Associate Professor in Structural Engineering, said: “The proposed research has the potential to revolutionise the way we assess our existing masonry infrastructure in the future and contribute towards network resilience and infrastructure sustainability not only in the UK but internationally”.
Although the study focused on the detection of cracks, future annotations of the masonry dataset could be updated to consider other defect types such as spalling (surface fatigue which leads to flaking and peeling), discoloration, and vegetation.
The outcomes of the deep learning approach used are heavily dependent on the quality of data. Thus, with an even more rich database, higher accuracy is likely to be achieved.
Further information
Codes, data and networks relevant to the study can be found in the GitHub repository: https://github.com/dimitrisdais/crack_detection_CNN_masonry