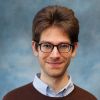
Massimiliano Fasi
- Position: Lecturer in Software Engineering
- Areas of expertise: Numerical Linear Algebra; Computer Arithmetic; Mathematical Software
- Email: M.Fasi@leeds.ac.uk
- Website: Googlescholar | ORCID
Profile
I obtained a bachelor’s degree in computer science (University of Perugia, Italy, 2012), and two master’s degree, one in theoretical computer science (École Normale Supérieure of Lyon, France, 2014), and one in computer science (University of Bologna, Italy, 2015). My most recent academic degree is a PhD in applied mathematics (University of Manchester, UK, 2019).
After receiving the degree, I was a postdoctoral research associate at the University of Manchester, UK, and at Örebro University, Sweden, where I held a fellowhip funded by the Wenner–Gren Foundations.
Before joining the University of Leeds, I was an Assistant Professor in Scientific Computing at Durham University, UK.
<h4>Research projects</h4> <p>Any research projects I'm currently working on will be listed below. Our list of all <a href="https://eps.leeds.ac.uk/dir/research-projects">research projects</a> allows you to view and search the full list of projects in the faculty.</p>Qualifications
- PhD in Applied Mathematics, University of Manchester
- MSc in Computer Science, University of Bologna
- MSc in Theoretical Computer Science, École normale supérieure de Lyon
- BSc in Computer Science, University of Perugia
Professional memberships
- Association for Computing Machinery (ACM)
- Internationa Linear Algebra Society (ILAS)
- National Group of Scientific Computing (INdAM GNCS)
- Society for Industrial and Applied Mathematics (SIAM)
- Unione Matematica Italiana (UMI)
Projects
-
<li><a href="//phd.leeds.ac.uk/project/1748-computer-arithmetic-for-the-next-generation-of-integrated-circuits">Computer Arithmetic for the Next Generation of Integrated Circuits</a></li>