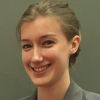
Zoe Hancox
- Email: ll15zlh@leeds.ac.uk
- Thesis title: Temporal Graph-Based Convolutional Neural Networks for Electronic Healthcare Record Data
Profile
Project Supervisors: Dr Samuel Relton, Dr Sarah Kingsbury, Professor Philip Conaghan, Professor Andrew Clegg
Zoe has been a Postgraduate Research Student on the UKRI Centre for Doctoral Training (CDT) in Artificial Intelligence for Medical Diagnosis and Care at The University of Leeds since 2020. Prior to joining this programme, Zoe’s previous degrees were in Medical Engineering, with projects developing tissue engineering methods to construct artificial organs to replace failing organs such as the cornea or breast tissue. She completed a BEng at The University of Bradford in Medical Engineering in 2019, followed by an MSc at The University of Leeds in Medical Engineering in 2020.
Research interests
Zoe’s research focuses on using a novel artificial intelligence technique involving temporal networks alongside electronic health record data to improve the predictive performance of early medical diagnosis. Timescales are not something greatly explored by GPs in the UK and temporal information is often difficult to implement into predictive tools, however, the hidden information found when connecting timing with symptoms can help better determine when worsening of an illness will occur. This time-symptom information could be used to enable early intervention before disease progression. Using this novel method could be hugely beneficial for predicting when and how best to treat a patient. Additionally, Zoe is exploring the impact of multimorbidity on patient trajectory to determine further risk factors. Predicting outcomes in a timely manner for patients with chronic conditions or cancer could reduce patient suffering, enhance treatment timings, and improve quality of life.
To find out more:
Qualifications
- MSc Medical Engineering, University of Leeds, 2020
- BEng Medical Engineering, University of Bradford, 2019