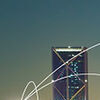
Sijia Li
- Email: mmsli@leeds.ac.uk
- Supervisors: Dr Luisa Cutillo, Dr Martín López-García
Profile
Sijia Li is an EPSRC-funded PhD candidate in Statistics and Postgraduate Researcher representative at University of Leeds. Sijia Li got her MSc degree in Financial Mathematics with Distinction from King’s College London in 2018, and a Bachelor degree in Mathematics and Applied Mathematics in 2017.
Sijia Li is currently working with Dr Cutillo and Dr López-García. She has developed a novel machine learning algorithm, Scalable Bigraphical Lasso, to infer the conditional dependency across both instances and features from high-dimensional count data. In the case of single cell RNA sequencing data, this method can be used to learn the gene regulatory network and the temporal structure of cells simultaneously. The algorithm solved the tensor-decomposition based problem 300 times faster than previous approaches while maintaining high accuracy.
Sijia Li has also investigated a simulation-based approach for sensitivity and reliability analysis for Bayesian Hierarchical Models (Bayesian Network).
As a result of her research, Sijia Li has got two first-author papers accepted into peer-reviewed top conferences in the field of data science and statistics, and she has presented her work at various international conferences.
Publications:
- Li Sijia, Martín López-García, Neil D. Lawrence, and Luisa Cutillo. “Two-way Sparse Network Inference with Count Data”, Proceedings of the Twenty-Fifth International Conference on Artificial Intelligence and Statistics. Preprint
- Li Sijia, Martín López-García, and Luisa Cutillo. "Simulation-based Evaluation of the Reliability of Bayesian Hierarchical Models for sc-RNAseq Data." 2021 20th International Conference on Ubiquitous Computing and Communications (IUCC/CIT/DSCI/SmartCNS). IEEE, 2021. Open-access version
Research interests
- Probabilistic Graph Model
- Tensor-decomposition
- Network Inference
- Fast Inference
- Bayesian Network
- Bio-Statistics
Qualifications
- MSc Financial Mathematics, King's College London
- BSc Mathematics and Applied Mathematics, Wuhan University