Solubility prediction problem addressed
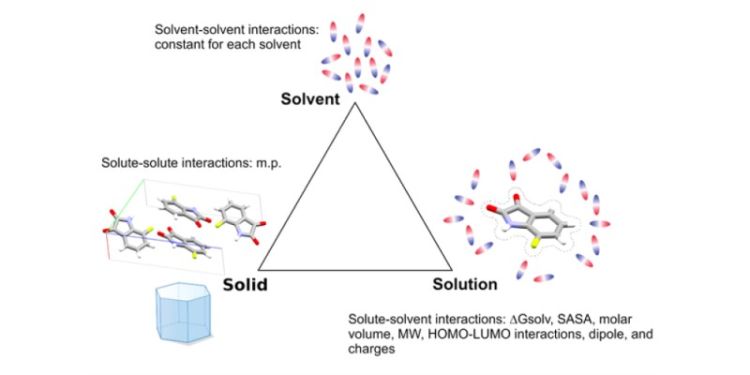
A landmark paper in solubility prediction in organic solvents and water by Leeds researchers has been published in Nature Communications.
The paper “Machine learning with physicochemical relationships: solubility prediction in organic solvents and water” has recently been published in Nature Communications.
Solubility prediction is a major challenge in chemical science and engineering, as it underpins progresses in in drug development, synthetic route and chemical process design, and high value product purification and crystallisation. Aqueous solubility prediction, in particular, has been the subject of the intensive research due to its biological relevance as well as importance in environmental and agrochemical predictions.
The paper reports a major step forward in improving solubility prediction using AI and Machine Learning.
The team at the University of Leeds, consisting of PhD student Samuel Boobier, Professor John Blacker (School of Chemical and Process Engineering), and Dr Bao Nguyen (School of Chemistry), have successfully delivered solubility prediction models in organic solvents and water with accuracy close to experimental errors.
This was accomplished by combining Artificial Intelligence and computational chemistry, using an approach called Causal Structure Property Relationship. The models outperformed established solubility prediction tools, e.g. COSMOTherm from Dassault Systemes, when validated against solubility data from AstraZeneca, the industrial partner in the project.
The lead author, Dr Bao Nguyen, said: “The paper reports a major step forward in improving solubility prediction using AI and Machine Learning. Our approach, which focuses on the interpretation of the physical and chemical aspects of dissolution process into a numeric problem, led to interpretable prediction models which reproduce the experimental dependence of solubility on solute-solute and solute-solvent interactions. Thus, we can rationally improve prediction accuracy by applying more accurate molecular modelling techniques to the right properties which we feed into the models. As things stand, we have reached the maximum accuracy allowed by the training data, and further improvement must come from the data itself. Ultimately these predictions will influence decision making in Research & Development, and establishing causality beyond simple correlations is an essential part in improving the confidence in our predictions.”
Further read: